Original Source: AIGC Open Community
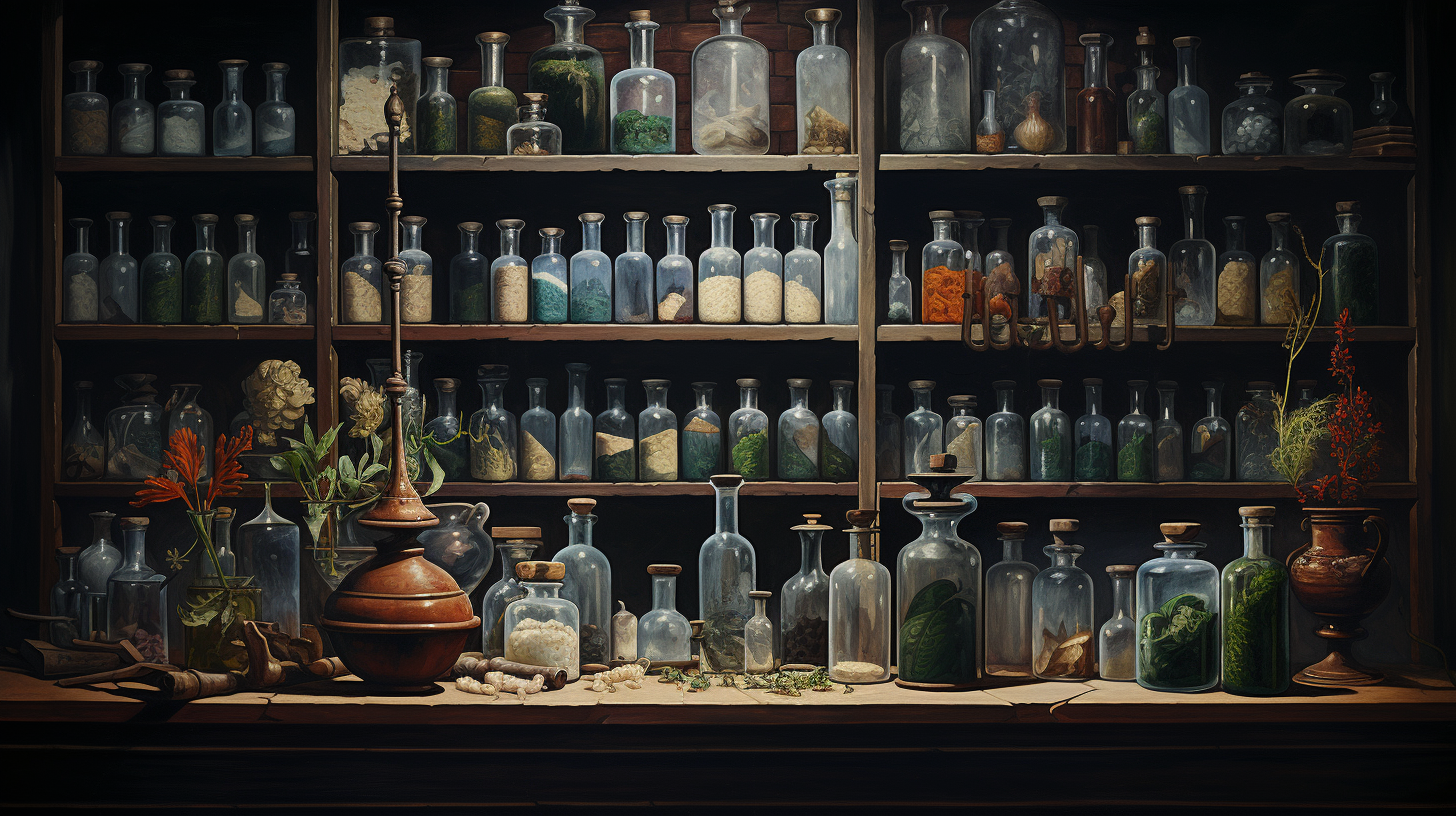
Image Source: Generated by Wujie AI
On November 1st, Google's AI research organization DeepMind announced on its official website the latest technological advancements of the protein structure prediction model AlphaFold: it has significantly improved prediction accuracy and expanded its coverage from proteins to other biological molecules, including ligands (small molecules).
It is reported that AlphaFold can predict almost all molecules in the Protein Data Bank (PDB), including ligands, proteins, nucleic acids (DNA and RNA), and molecules with post-translational modifications (PTMs), achieving atomic-level laboratory-grade accuracy, which is crucial for medical research.
AlphaFold Protein Structure Database: https://alphafold.ebi.ac.uk/
Latest Progress: https://storage.googleapis.com/deepmind-media/DeepMind.com/Blog/a-glimpse-of-the-next-generation-of-alphafold/alphafold_latest_oct2023.pdf
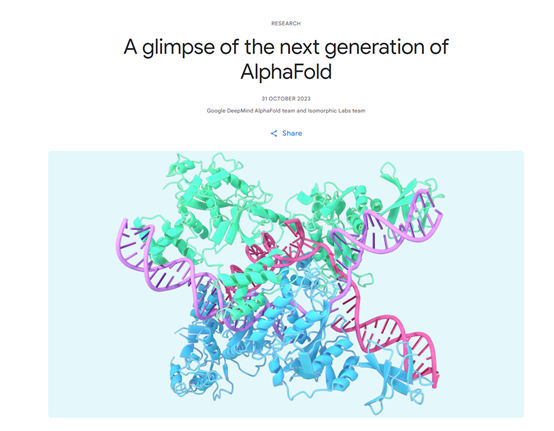
Beyond Protein Folding
Since Google first released AlphaFold in 2020, it has fundamentally changed the understanding of proteins and their interactions, successfully predicting the three-dimensional structure of proteins and helping achieve major technological breakthroughs in the field of bioscience.
AlphaFold achieved a fundamental breakthrough in the prediction of single-chain protein structures. Then AlphaFold-Multimer expanded to include complexes with multiple protein chains, followed by AlphaFold2.3, which improved performance and expanded coverage to larger complexes.
In 2022, AlphaFold's structure predictions covered almost all cataloged proteins known to science, and these predictions, in collaboration with the European Bioinformatics Institute (EMBL-EBI), have been made freely available in the AlphaFold Protein Structure Database.
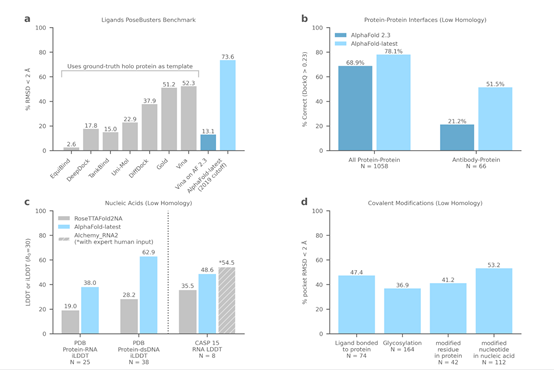
So far, over 1.4 million users from more than 190 countries have accessed the AlphaFold Protein Structure Database, and scientists from around the world have used AlphaFold's predictions to advance research, from accelerating the development of new malaria vaccines and advancing cancer drug discovery to studying enzymes for plastic degradation to address pollution.
The latest AlphaFold model is not limited to protein folding but can also generate highly accurate structure predictions for ligands, proteins, nucleic acids, and post-translational modifications.
Accelerating Drug Development
Data analysis shows that the latest AlphaFold model significantly outperforms AlphaFold2.3 in some protein structure prediction problems related to drug discovery, such as antibody binding. In addition, accurate prediction of protein and ligand structures is an important tool for drug discovery, helping scientists identify and design new molecules that may become drugs.
The current standard in the pharmaceutical industry is to use "docking methods" to determine the interaction between ligands and proteins. These docking methods require a rigid reference protein structure and a proposed binding position for the ligand.
The latest AlphaFold model sets a new standard for protein-ligand structure prediction, surpassing the best reported docking methods, without requiring a reference protein structure or the position of the ligand pocket, allowing predictions for entirely new proteins that have never been structurally characterized before.
It can also jointly model the positions of all atoms, allowing it to represent the full inherent flexibility of proteins and nucleic acids when interacting with other molecules, something docking methods cannot achieve.
For example, here are three recent cases closely matching the structures predicted by the latest AlphaFold model (shown in color) with experimentally determined structures (shown in gray), all with therapeutic relevance:
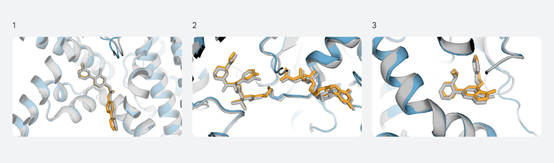
Predictions for PORCN (1), KRAS (2), and PI5P4Kγ (3)
PORCN: A clinical-stage anti-cancer molecule bound to its target and another protein.
KRAS: A trimeric complex formed with a covalent ligand (a molecular glue) targeting an important cancer target.
PI5P4Kγ: A selective lipid kinase modulator with implications for multiple diseases, including cancer and immune disorders.
A New Understanding of Biology
By unlocking the modeling of protein and ligand structures, as well as nucleic acids and structures with post-translational modifications, the latest AlphaFold model provides a faster and more accurate tool for examining basic biology.
For example, the structure involving CasLambda binding with crRNA and DNA, which is part of the CRISPR family.
CasLambda shares the gene editing ability with the widely known "gene scissors" CRISPR-Cas9 system, which researchers can use to alter the DNA of animals, plants, and microbes. The smaller size of CasLambda may make it more efficient for gene editing.
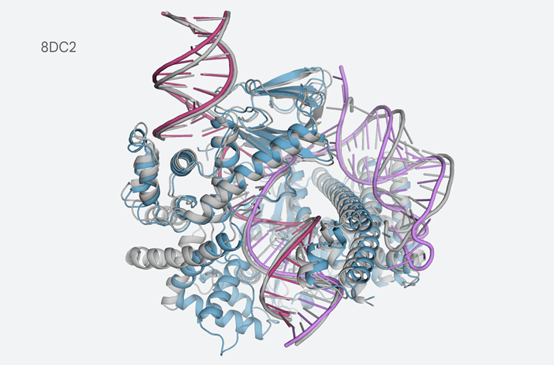
The predicted structure of CasLambda (Cas12l) binding with crRNA and DNA (part of the CRISPR subsystem)
AlphaFold is capable of modeling such complex systems, indicating that AI models can help the medical and biological community better understand these types of mechanisms and accelerate their application in therapy.
Google DeepMind stated that the latest AlphaFold model can help achieve biomedical breakthroughs and build the next "digital biology" era, providing crucial detailed predictions for genomics, bio-renewable materials, plant immunity, potential therapeutic targets, drug design, and more.
免责声明:本文章仅代表作者个人观点,不代表本平台的立场和观点。本文章仅供信息分享,不构成对任何人的任何投资建议。用户与作者之间的任何争议,与本平台无关。如网页中刊载的文章或图片涉及侵权,请提供相关的权利证明和身份证明发送邮件到support@aicoin.com,本平台相关工作人员将会进行核查。