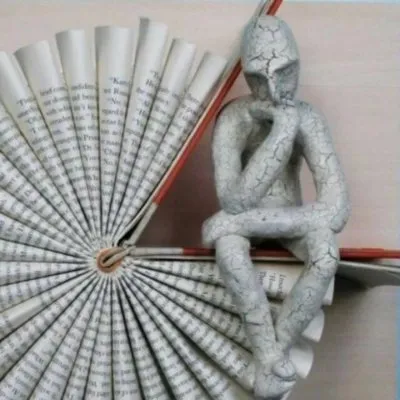
Haotian | CryptoInsight|May 21, 2025 06:51
This research report by @ LazAINetwork raises an interesting topic: if our target AI+web3 industry experiences DeFi like summer moments, then the AI industry currently lacks standardized value measurement systems such as TVL, APY, Liquidity, etc. We cannot evaluate AI value solely based on Mindshare (MEME), right? How to do it specifically? Let me share my opinion along with this report:
1) The true birth of DeFi Summer is the emergence of a bunch of quantifiable data indicators such as TVL, APY, and liquidity. TVL tells you 'how much capital is involved', APY tells you 'how much profit can be earned', and liquidity indicates' how convenient trading is'.
These indicators enable institutional funds and individual investors to clearly understand the value of a DeFi protocol, thus driving explosive growth from zero to billions of dollars.
On the other hand, in the current AI Agent race, what is used to measure the value of an AI project? Financing scale? Model parameter quantity? Training costs? Subscription users? Obviously, the reality is that data contributors cannot see how much value their annotated data is worth, model developers do not know how much value their models have created, and the fine tuned models have almost lost the ability to trace their origins, making it difficult to track their value.
The entire AI race track is more like a huge 'black box' of chaotic states, knowing that there is a lot of value flowing, but it cannot be effectively quantified and allocated. So LazAI is trying to define a set of value standards for AI similar to "TVL", but its TVL is not locked in funds, but a new standard for defining AI native assets such as "data value", "model performance", "agent behavior", etc.
2) So the question is, LazAI's redefined AI value quantification system mainly includes three key mechanisms:
1. DAT (Data Anchored Token): can be understood as the "digital identity card" of data. Traditional NFTs can only prove that you have a boring ape image, while DAT not only records ownership, but also fully records the entire "life cycle" of the data: who created it? Which models have been used? What effect did it produce? All of this information is uploaded to the blockchain, forming a verifiable resume.
More importantly, DAT directly assists in data pricing, transforming data from intangible resources into assets with price tags. As a DAT is used by more models and generates higher value, its inherent value will naturally increase. For example, a set of professionally annotated medical data may initially be worth $10 per piece, but when it proves to improve model accuracy by 5%, its value may increase to $50 per piece;
2. IDAO (Individual Center Autonomous Organization): It is an innovative mechanism for AI governance. Each data contributor or AI agent is an independent decision-making unit. Imagine that you are no longer a passive data provider, but a "small DAO" that can actively set its own data usage rules.
In other words, iDAO makes AI systems programmable. You can set rules: for every time my medical imaging data is used by the AI diagnostic system, how much profit do I receive; My writing materials can only be used for specific types of creative AI. This directly establishes a direct connection between data contribution and value return, eliminating the need for platforms to act as intermediaries;
3. Verifiable computing framework: Current AI models are like "black boxes", where does the training data come from? How is the reasoning process? What is the credibility of the results? These questions lack transparent answers. The verifiable computing framework requires AI behavior to be traceable throughout the entire process: data sources, processing methods, inference paths, and result reliability, all of which can be verified on chain.
This framework ensures the authenticity of DAT, the enforceability of iDAO decisions, and the accountability of AI behavior. Simply put, it has transformed AI from a 'trust game' into a 'chain of evidence', providing a foundation of trust for the entire economic system.
3) After having the above three sets of AI quantifiable standard systems, how can they be transformed into an AI version of "TVL"?
Taking a common medical scenario as an example: Suppose an AI agent has 500 complete medical records corresponding to treatment plans, with a single value of $200, its total value can be quantified as $100000. At the same time, these data will generate model performance indices when applied to the training process. For example, based on these raw data, the accuracy of diagnosing a certain disease can reach 95%; Then this AI system will also generate utility scores in continuous clinical applications, such as doctor adoption rate, historical diagnostic accuracy, and so on.
These data dimensions will be recorded and verifiable on the chain throughout the entire process, including how much high-quality data (TDVL) is concatenated, how many high-performance models are generated (model performance index), and how many valuable applications are generated (proxy utility). This is the AI version of 'TVL'.
4) In fact, this set of value evaluation standards will have a self reinforcing multi-party win-win incentive system, where data contributors (such as doctors, financial analysts, legal experts) manage their professional data through iDAO and receive profit sharing every time the data is used; Model developers can obtain high-quality data, build more accurate models, and provide better services; End users can choose the most suitable AI service based on transparent performance indicators; Validators receive rewards by confirming the authenticity of data, model performance, and agent behavior.
When the contribution of data directly equals the economic return, the market will spontaneously generate various high-quality vertical domain datasets; When the model performance has objective quantitative indicators, innovation will receive fair market testing;
This multi-party win-win model will give rise to a new "AI native economy", just as DeFi has transparent indicators such as TVL and APY to create a flywheel of "locking assets → gaining profits → attracting more assets → ecological growth". With this new set of quantitative indicators, AI is no exception.
above.
From a long-term perspective, the development trend of the AI industry will evolve from general models to vertical specialization, and a set of quantifiable data value standards will become a necessity for this trend evolution, perhaps becoming the "DeFi Moment" that ignites the AI Agent race track
Share To
HotFlash
APP
X
Telegram
CopyLink