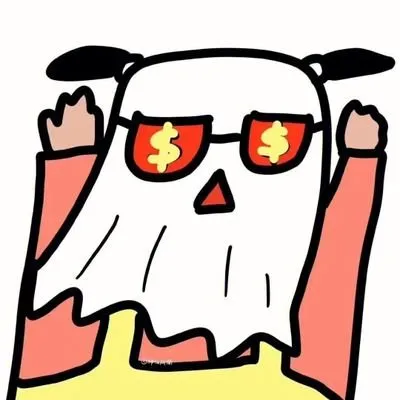
看不懂的sol|May 06, 2025 15:02
Do you really understand what quantitative trading is?
Traditional Trading: Experienced Fishermen Determine Where to Find Fish (See K-line Chart/Listen to News)
Quantitative trading: using sonar to scan the seabed terrain (mathematical model), automatic network deployment and collection (programmatic trading)
The advantages of quantitative trading include discipline, systematicity, real-time performance, and decentralization.
Shortcomings of quantitative trading include sample error and bias, strategy resonance, incorrect attribution, black box, etc.
Why is quantitative trading necessary?
Traditional investment methods often rely on experience and intuition, but people are easily influenced by emotions such as panic and greed, and do not manage assets based on personal feelings.
Quantitative trading analyzes large amounts of data and models to identify market patterns, reduce subjective interference, find investment targets, establish reusable and optimized investment strategies, and guide the investment decision-making process.
In terms of application, quantitative investment almost covers the entire investment process, including quantitative stock selection, quantitative timing, stock index futures arbitrage, commodity futures arbitrage, statistical arbitrage, algorithmic trading, asset allocation, risk control, and of course, the cryptocurrency market.
02. Advantages of Quantitative Trading
Disciplinary nature
Traditional investment is largely influenced by emotions such as greed and fear, and sometimes it is difficult to ensure discipline in trading execution.
Generally, the investment instructions given by quantitative models are strictly followed, and will not be changed arbitrarily with changes in investor sentiment, thus having relatively strict trading discipline.
systematic
The systematic characteristics of quantification mainly include multi-level quantification models, multi angle observations, and massive data processing.
The multi-level model mainly includes asset allocation models, industry selection models, and selected individual stock models.
Multi angle observation mainly includes analysis of macro cycles, market structure, corporate valuation, growth and profitability quality, market sentiment, and other multiple perspectives.
Massive data processing refers to the ability of quantitative investment to obtain data and information processing capabilities far beyond the human brain through computers, thereby capturing more investment opportunities.
real-time
Being able to track market changes in a timely and rapid manner, constantly discovering new statistical models that can provide excess returns, and seeking new trading opportunities. Quantification is constantly searching for valuation pitfalls, capturing opportunities brought about by mispricing and overvaluation through comprehensive and systematic scanning.
decentralization
The diversification of quantitative trading can also be said to rely on probability to win.
This manifests in two aspects:
One is to discover patterns from historical data, which are often strategies that had a high probability of winning in the past;
The second is to rely on selecting a combination of stocks/currencies to win, rather than winning with just one or a few stocks/currencies. From the perspective of investment portfolio philosophy, it is also to capture stocks/currencies with a high probability of winning, rather than betting on a single stock/currency.
03. Shortcomings of Quantitative Trading
Sample error and sample bias
Many quantitative strategies heavily rely on historical data, but historical data may lack sufficient diversity and accumulation over a long period of time, resulting in errors in sample sampling due to insufficient quantity or biases due to non random sampling.
The correlation rules obtained on this basis may become invalid and lose their referenceability once they leave the sample range.
Strategic resonance
Many quantitative strategies, similar to technical analysis strategies, once a strategy is proven effective, its effectiveness weakens as the number of users increases and the strategy resonates.
Wrong attribution
In the widely used multi factor quantization strategy, the cause is inferred from the results of the data. As long as enough factors are constructed, it is likely to achieve a specific known result.
However, when a quantitative strategy based on this multi factor combination is used for actual trading, it may fail due to incorrect attribution. Because it is difficult to accurately distinguish between accidental factors and decisive causal factors by regressing from the results.
black box
Various quantitative strategies, including high-frequency, hedging, or arbitrage, often have no inherent causal relationship, and their effectiveness is mostly based on the strong correlation of historical data. The logic of the strategy is that based on historical data, if there is a 55% or higher probability that it is effective, then as long as there is enough duplicate data, the chances of winning will accumulate.
How does quantitative trading work?
collect data
Collect historical data on financial products such as stocks, currencies, bonds, futures, etc., including prices, trading volumes, and company financial statements.
development model
According to the data, patterns are discovered, such as "a certain coin frequently rises after 3 pm, trading orders, pending orders, funding rates, etc. Transform these laws into mathematical models, such as certain formulas or rules.
Backtesting strategy
Use historical data to test whether these rules are effective and see if you can make money using this method in the past.
Execute transaction
Use computer programs to automate the execution of transactions, such as placing orders automatically when rules are found to be valid.
There are two approaches to building strategies
One is data mining, which discovers stable structures through statistics and induction in a pile of data, and commonly used techniques for analysis are of this type. Due to the random fluctuations in price data, it is unlikely to have a sustained and stable structure, requiring continuous iterative optimization. However, with limited new data generated in the future, it is difficult to discover new stable structures within a small amount of data. Therefore, once the statistical patterns of historical data fail, the strategy loses its value.
The development path of this strategy is to first have data, then explore patterns, and continuously optimize and iterate.
The second type is logical reasoning, which derives a conclusion through mathematical deduction, such as the parity arbitrage theory. Through calculation, an arbitrage boundary is determined, and as long as the price exceeds the boundary, there is an arbitrage opportunity. No matter how the price changes, as long as it exceeds the arbitrage boundary, there is a arbitrage opportunity.
The development path of this strategy is to first logically derive rules, and then select proxies for basic conditions, such as changes in interest rates and storage costs, to obtain different calculation results. The new calculation results are then used to wait for price triggered trading opportunities.
Tomorrow I will release a video to see how top Wall Street traders use quantitative arbitrage to generate billions!
Share To
HotFlash
APP
X
Telegram
CopyLink